npj:高居里温度2D铁磁材料的快速筛选—高通量计算的威力

海归学者发起的公益学术平台
分享信息,整合资源
交流学术,偶尔风月
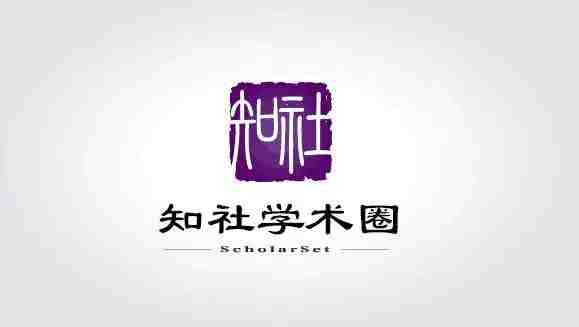
来自印度科学院(IISc)电子系统工程系纳米器件研究实验室Arnab Kabiraj团队开发了一个程序,该程序执行基于第一原理的计算,然后进行基于海森堡模型的蒙特卡罗模拟,以从任何磁性二维材料晶体结构准确预测居里点。基于此代码的程序能够以高通量的方式执行计算,使用此代码能够从合适的数据库确定材料的居里点。令人惊讶的是,786种材料中有47%被归为FM,成功地测定了157种材料的TC 等磁性能。对于一些材料,预测结果与实验测量的TC 非常接近,验证了高通量方法的准确性。为了更快地发现高TC材料,进一步开发了使用这157个数据点的机器学习(ML)流水线。使用这个ML模型,可以从文献和其他数据库中识别出一些高居里温度的2DFM材料。本方法采用的信息学在准确性和效率之间达到了最佳平衡,提供了前所未有的机会来比较大量具有不同结构的材料的磁性,这可能会导致对二维磁性的许多新的见解。本研究证明了机器学习模型可以非常精确地捕捉与温度有关的自旋翻转的复杂过程。因此,可以大大提升计算材料的效率,并以促进二维磁性的快速筛选和实际应用。
该文近期发表于npj Computational Materials 6: 35 (2020),英文标题与摘要如下,点击左下角“阅读原文”可以自由获取论文PDF。

High-throughput discovery of high Curie point two-dimensional ferromagnetic materials
Arnab Kabiraj, Mayank Kumar and Santanu Mahapatra
Databases for two-dimensional materials host numerous ferromagnetic materials without the vital information of Curie temperature since its calculation involves a manually intensive complex process. In this work, we develop a fully automated, hardwareaccelerated, dynamic-translation based computer code, which performsfirst principles-based computations followed by Heisenberg model-based Monte Carlo simulations to estimate the Curie temperature from the crystal structure. We employ this code to conduct a high-throughput scan of 786 materials from a database to discover 26 materials with a Curie point beyond 400 K. For rapid data mining, we further use these results to develop an end-to-end machine learning model with generalized chemical features through an exhaustive search of the model space as well as the hyperparameters. We discover a few more high Curie point materials from different sources using this data-driven model. Such material informatics, which agrees well with recent experiments, is expected to foster practical applications of two-dimensional magnetism.

本文系网易新闻·网易号“各有态度”特色内容
媒体转载联系授权请看下方
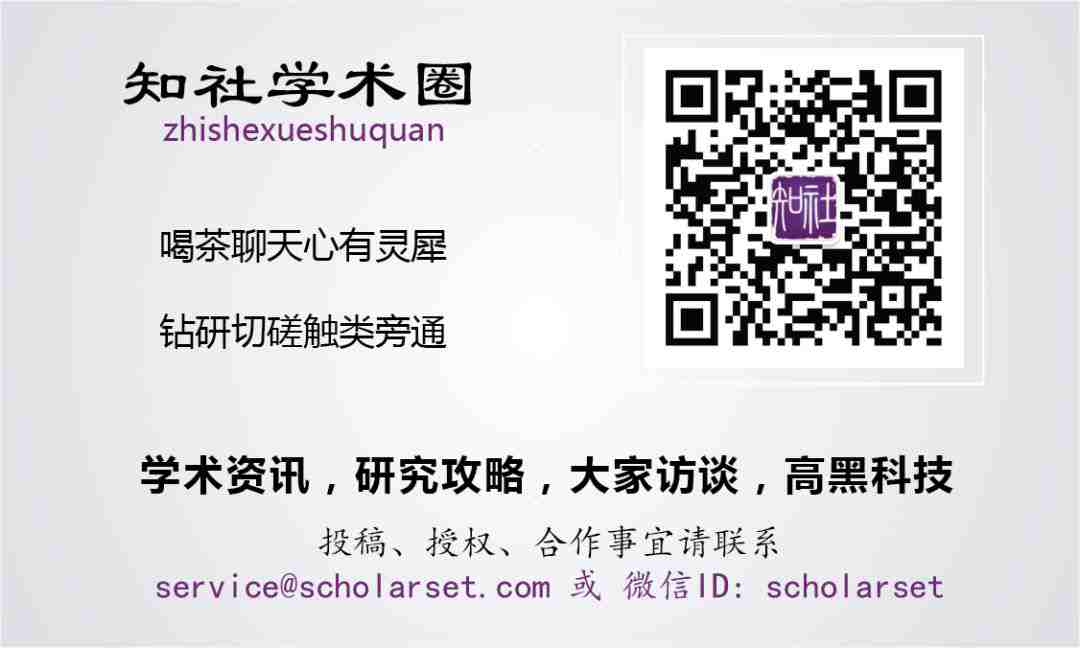
关键词
网易
磁性
结构
方法
温度
最新评论
推荐文章
作者最新文章
你可能感兴趣的文章
Copyright Disclaimer: The copyright of contents (including texts, images, videos and audios) posted above belong to the User who shared or the third-party website which the User shared from. If you found your copyright have been infringed, please send a DMCA takedown notice to [email protected]. For more detail of the source, please click on the button "Read Original Post" below. For other communications, please send to [email protected].
版权声明:以上内容为用户推荐收藏至CareerEngine平台,其内容(含文字、图片、视频、音频等)及知识版权均属用户或用户转发自的第三方网站,如涉嫌侵权,请通知[email protected]进行信息删除。如需查看信息来源,请点击“查看原文”。如需洽谈其它事宜,请联系[email protected]。
版权声明:以上内容为用户推荐收藏至CareerEngine平台,其内容(含文字、图片、视频、音频等)及知识版权均属用户或用户转发自的第三方网站,如涉嫌侵权,请通知[email protected]进行信息删除。如需查看信息来源,请点击“查看原文”。如需洽谈其它事宜,请联系[email protected]。