npj:扫描探针显微镜插上机器学习的翅膀——材料探幽更为便捷

海归学者发起的公益学术平台
分享信息,整合资源
交流学术,偶尔风月
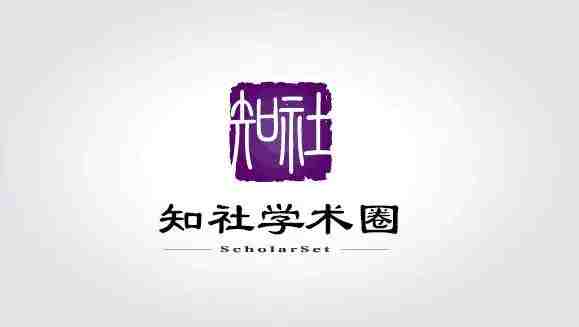
因此,有限数据构成的数据集只能算“小”数据,这导致高阶统计方法失效,无监督学习方法的选择仅限于利用低阶统计的方法。扫描探针显微镜的功能成像模式,可测量外部因素(如温度或磁场)变化导致的材料性质变化。来自德国杜伊斯堡-埃森大学和葡萄牙阿威罗大学的Harsh Trivedi及其同事,以此作为一个有趣的“小”数据案例,报道了采用可变磁场压电响应力显微镜(PFM)对多铁复合材料的研究,其中压电响应的磁场依赖性,因实验限制而采取粗步长测量;他们基于密度聚类(DBSCAN)和主成分分析算法(PCA)所得到的关键特征,成功地反映了两种材料之间的磁电响应差异,从而证明了运用无监督机器学习技术能从较少的测量结果中提取重要规律。这一技术方便又成本低廉,有望在功能材料的分析中得到更广泛的应用。
该文近期发表于npj Computational Materials 4: 28 (2018) ,英文标题与摘要如下,点击左下角“阅读原文”可以自由获取论文PDF。

Sequential piezoresponse force microscopy and the ‘small-data’ problem
Harsh Trivedi, Vladimir V. Shvartsman, Marco S. A. Medeiros, Robert C. Pullar & Doru C. Lupascu
The term big-data in the context of materials science not only stands for the volume, but also for the heterogeneous nature of the characterization data-sets. This is a common problem in combinatorial searches in materials science, as well as chemistry. However, these data-sets may well be ‘small’ in terms of limited step-size of the measurement variables. Due to this limitation, application of higher-order statistics is not effective, and the choice of a suitable unsupervised learning method is restricted to those utilizing lower-order statistics. As an interesting case study, we present here variable magnetic-field Piezoresponse Force Microscopy (PFM) study of composite multiferroics, where due to experimental limitations the magnetic field dependence of piezoresponse is registered with a coarse step-size. An efficient extraction of this dependence, which corresponds to the local magnetoelectric effect, forms the central problem of this work. We evaluate the performance of Principal Component Analysis (PCA) as a simple unsupervised learning technique, by pre-labeling possible patterns in the data using Density Based Clustering (DBSCAN). Based on this combinational analysis, we highlight how PCA using non-central second-moment can be useful in such cases for extracting information about the local material response and the corresponding spatial distribution

今日福利:免费攻读人工智能专业,限时免费,扫二维码参与课程学习

扩展阅读
本文系网易新闻·网易号“各有态度”特色内容
媒体转载联系授权请看下方
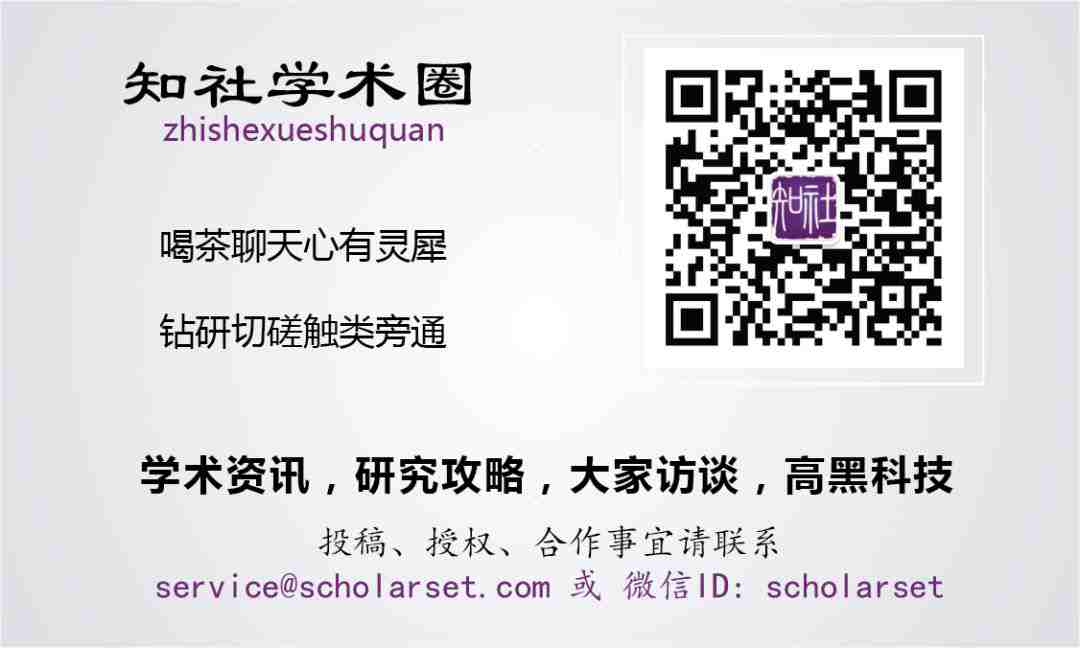
最新评论
推荐文章
作者最新文章
你可能感兴趣的文章
Copyright Disclaimer: The copyright of contents (including texts, images, videos and audios) posted above belong to the User who shared or the third-party website which the User shared from. If you found your copyright have been infringed, please send a DMCA takedown notice to [email protected]. For more detail of the source, please click on the button "Read Original Post" below. For other communications, please send to [email protected].
版权声明:以上内容为用户推荐收藏至CareerEngine平台,其内容(含文字、图片、视频、音频等)及知识版权均属用户或用户转发自的第三方网站,如涉嫌侵权,请通知[email protected]进行信息删除。如需查看信息来源,请点击“查看原文”。如需洽谈其它事宜,请联系[email protected]。
版权声明:以上内容为用户推荐收藏至CareerEngine平台,其内容(含文字、图片、视频、音频等)及知识版权均属用户或用户转发自的第三方网站,如涉嫌侵权,请通知[email protected]进行信息删除。如需查看信息来源,请点击“查看原文”。如需洽谈其它事宜,请联系[email protected]。