npj:机器学习——小规模数据集也能用来预测

海归学者发起的公益学术平台
分享信息,整合资源
交流学术,偶尔风月
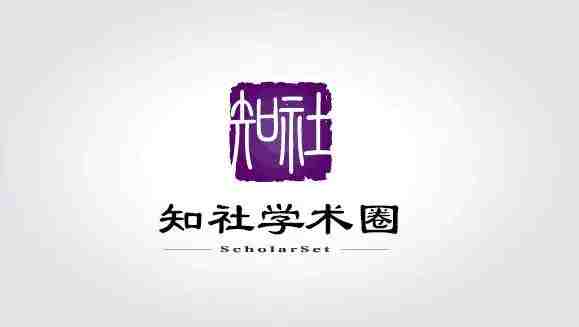
有证据表明,机器学习的预测能力与其可用的数据集的规模成正比。相比其他领域,材料的数据集规模更小且更多样化,这就给机器学习应用于新材料和新属性的预测带来了麻烦——小规模数据集意味着预测准确性不高。来自北美丰田研究院的Ying Zhang和Chen Ling分析了材料数据集的可用性与机器学习模型预测能力之间的关系,找到了问题的核心:小规模数据集影响算法的自由度,从而约束其准确预测能力。他们找到了解决问题的办法:用其他方式对感兴趣的性质作粗略估算,再将获得的估算结果引入代码。他们在预测二元半导体带隙、晶格热导率和沸石弹性特性的三个研究案例中,通过引入粗略估算有效地提高了机器学习模型的预测能力,达到最优水平。该结果表明,他们提出的利用小规模材料数据集构建精确机器学习模型的策略具有通用性。该文近期发表于npj Computational Materials 4: 25 (2018); doi:10.1038/s41524-018-0081-z。英文标题与摘要如下,点击阅读原文可以自由获取论文PDF。

A strategy to apply machine learning to small datasets in materials science
Ying Zhang & Chen Ling
There is growing interest in applying machine learning techniques in the research of materials science. However, although it is recognized that materials datasets are typically smaller and sometimes more diverse compared to other fields, the influence of availability of materials data on training machine learning models has not yet been studied, which prevents the possibility to establish accurate predictive rules using small materials datasets. Here we analyzed the fundamental interplay between the availability of materials data and the predictive capability of machine learning models. Instead of affecting the model precision directly, the effect of data size is mediated by the degree of freedom (DoF) of model, resulting in the phenomenon of association between precision and DoF. The appearance of precision–DoF association signals the issue of underfitting and is characterized by large bias of prediction, which consequently restricts the accurate prediction in unknown domains. We proposed to incorporate the crude estimation of property in the feature space to establish ML models using small sized materials data, which increases the accuracy of prediction without the cost of higher DoF. In three case studies of predicting the band gap of binary semiconductors, lattice thermal conductivity, and elastic properties of zeolites, the integration of crude estimation effectively boosted the predictive capability of machine learning models to state-of-art levels, demonstrating the generality of the proposed strategy to construct accurate machine learning models using small materials dataset.

本文系网易新闻·网易号“各有态度”特色内容
媒体转载联系授权请看下方
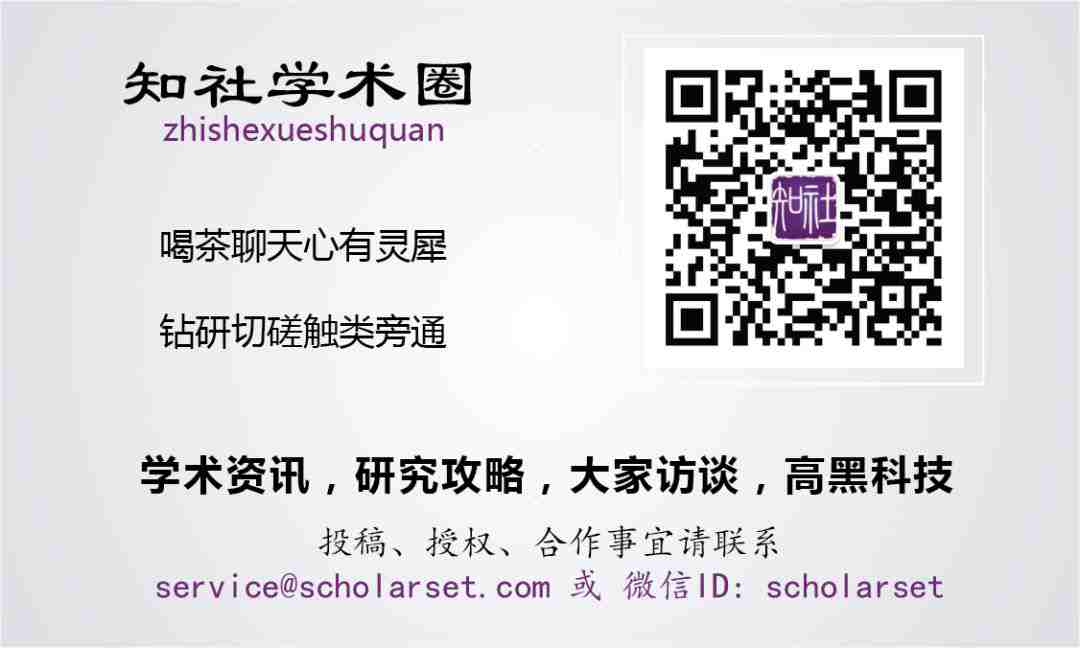
最新评论
推荐文章
作者最新文章
你可能感兴趣的文章
Copyright Disclaimer: The copyright of contents (including texts, images, videos and audios) posted above belong to the User who shared or the third-party website which the User shared from. If you found your copyright have been infringed, please send a DMCA takedown notice to [email protected]. For more detail of the source, please click on the button "Read Original Post" below. For other communications, please send to [email protected].
版权声明:以上内容为用户推荐收藏至CareerEngine平台,其内容(含文字、图片、视频、音频等)及知识版权均属用户或用户转发自的第三方网站,如涉嫌侵权,请通知[email protected]进行信息删除。如需查看信息来源,请点击“查看原文”。如需洽谈其它事宜,请联系[email protected]。
版权声明:以上内容为用户推荐收藏至CareerEngine平台,其内容(含文字、图片、视频、音频等)及知识版权均属用户或用户转发自的第三方网站,如涉嫌侵权,请通知[email protected]进行信息删除。如需查看信息来源,请点击“查看原文”。如需洽谈其它事宜,请联系[email protected]。