npj:从头探索和自导学习—势能面

海归学者发起的公益学术平台
分享信息,整合资源
交流学术,偶尔风月
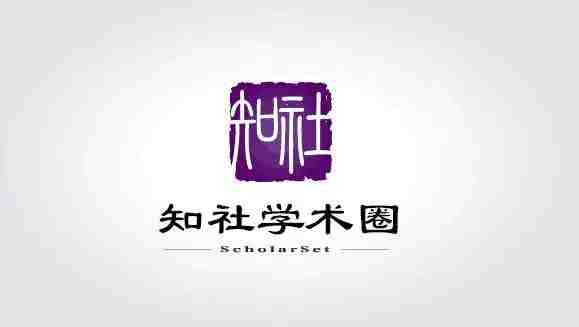
为解决这一老大难问题,人们将目光转向了机器学习(ML)。基于ML的新方法通过拟合具有DFT计算精度的参考数据库来近似计算高维势能面(PES),并精确模拟ML原子间势,同时其模拟速度比传统DFT方法快几个数量级。ML原子间势可以解决具有挑战性的、涉及模拟数千个原子的复杂构型问题,如已实现的无定形碳膜的原子级沉积和生长、质子转移机制、材料中的位错探索等。新近的研究表明,ML原子间势可能是针对晶体相、簇和纳米结构进行整体结构搜索的合适工具。可以说用ML途径解决上述老大难问题前景一片光明,但目前,组建适合ML原子间势的参考数据库还非常局限,需要通过大量手动操作和人工调优的方法来创建,因此急需改变这一繁杂的操作模式。
该研究提出了一种高效且统一的方法,用于生成适合机器学习(ML)原子间势的参考数据库,通过ML驱动的搜索和相似性度量来探索构型空间,所有这些都不需事先知道哪些结构是相关或不相关的。由英国剑桥大学工程系和牛津大学化学系的Volker L. Deringer教授领导的研究小组揭示,ML原子间势可以以一种高度自动化的方式构建,并从一开始(从头开始)在同一协议中探索和拟合不同的势能面。与连续的主动学习相反,它们的目标是收敛并形成一个合理的势函数,即在无需额外拟合的情况下,这些势函数可以描述好各种构型。作者证明了该方法可以描述好从石墨片到密排过渡金属等多种结构和化合物。这项研究工作为计算机如何仅基于数据和相似性测量来获得结构化学提供了概念性的认知,并为在材料发现中更常规地应用ML势函数铺平了道路。
该文近期发表于npj Computational Materials 5: 99 (2019),英文标题与摘要如下,点击左下角“阅读原文”可以自由获取论文PDF。

De novo exploration and self-guided learning of potential-energy surfaces
Noam Bernstein, Gábor Csányi & Volker L. Deringer
Interatomic potential models based on machine learning (ML) are rapidly developing as tools for material simulations. However, because of their flexibility, they require large fitting databases that are normally created with substantial manual selection and tuning of reference configurations. Here, we show that ML potentials can be built in a largely automated fashion, exploring and fitting potential-energy surfaces from the beginning (de novo) within one and the same protocol.The key enabling step is the use of a configuration-averaged kernel metric that allows one to select the few most relevant and diverse structures at each step.The resulting potentials are accurate and robust for the wide range of configurations that occur during structure searching, despite only requiring a relatively small number of single-point DFT calculations on small unit cells.We apply the method to materials with diverse chemical nature and coordination environments, marking an important step toward the more routine application of ML potentials in physics, chemistry, and materials science.

本文系网易新闻·网易号“各有态度”特色内容
媒体转载联系授权请看下方
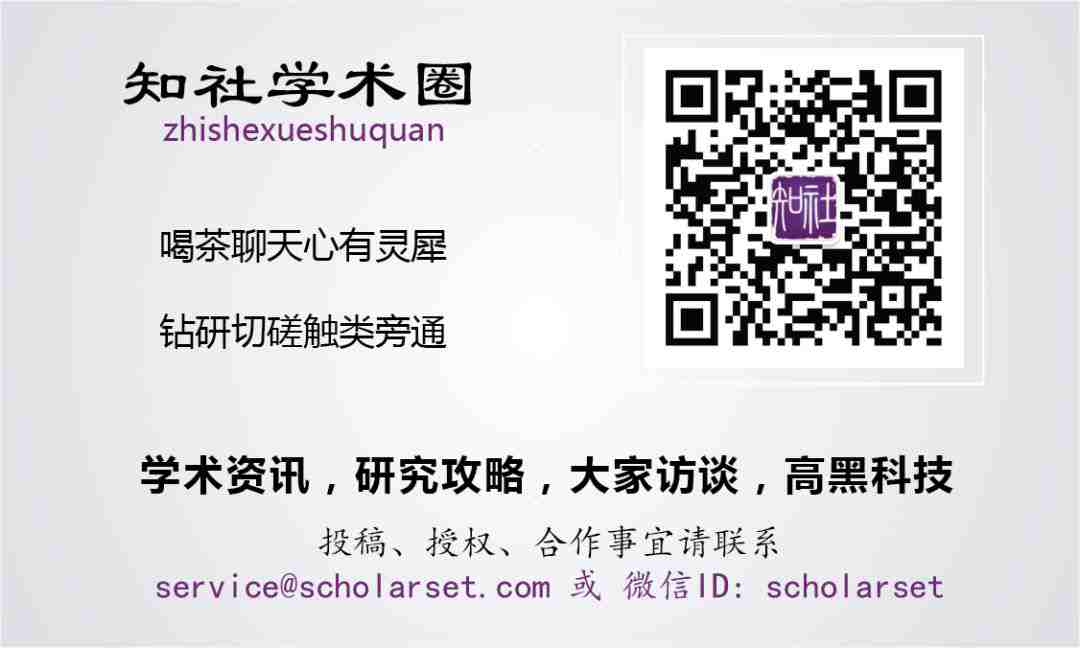
最新评论
推荐文章
作者最新文章
你可能感兴趣的文章
Copyright Disclaimer: The copyright of contents (including texts, images, videos and audios) posted above belong to the User who shared or the third-party website which the User shared from. If you found your copyright have been infringed, please send a DMCA takedown notice to [email protected]. For more detail of the source, please click on the button "Read Original Post" below. For other communications, please send to [email protected].
版权声明:以上内容为用户推荐收藏至CareerEngine平台,其内容(含文字、图片、视频、音频等)及知识版权均属用户或用户转发自的第三方网站,如涉嫌侵权,请通知[email protected]进行信息删除。如需查看信息来源,请点击“查看原文”。如需洽谈其它事宜,请联系[email protected]。
版权声明:以上内容为用户推荐收藏至CareerEngine平台,其内容(含文字、图片、视频、音频等)及知识版权均属用户或用户转发自的第三方网站,如涉嫌侵权,请通知[email protected]进行信息删除。如需查看信息来源,请点击“查看原文”。如需洽谈其它事宜,请联系[email protected]。