npj:电场响应材料数据库—多重高通量计算和机器学习

海归学者发起的公益学术平台
分享信息,整合资源
交流学术,偶尔风月
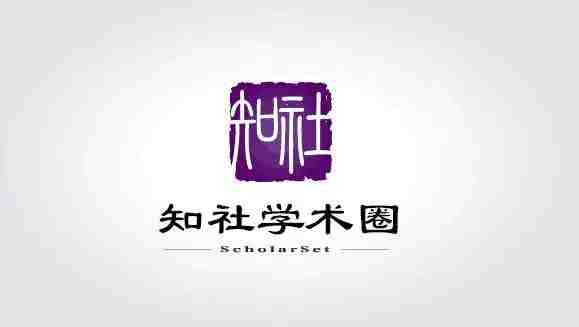
一个由美国国家标准局领导的研究团队针对JARVIS-DFT 计算材料数据库开展了基于密度泛函微扰理论的高通量计算。经过初步筛选,他们从约4万种材料中筛选了5千种材料,高通量计算了这些材料的红外吸收谱、介电和压电系数,由此构建了相关数据库。通过计算,他们发现了已有实验尚未报道过的部分高性能材料。基于该数据库,他们分析了材料各种特征,如维度、空间群及化学组成等与上述性能的关系,并得到了一些有意思的趋势。例如,部分氢氧化物具有高的红外振动模,而卤化物具有较低的红外振动模;具有正交晶格的氧化物具有较大压电系数;压电应变和压力张量间没有显著关联;带隙和介电系数具有反相关性;卤化物具有较低的介电系数,而氧化物具有较高的介电系数等。在这些定性分析的基础上,作者进一步采用了机器学习模型,建立了精确预测材料波恩电荷和红外振动模,以及高压电、介电材料分类的方法。该研究所建立的数据库,以及建立的定量和定性预测模型,将为高性能红外、介电和压电材料的筛选提供有力工具。
该文近期发表于npj Computational Materials 6: 64 (2020),英文标题与摘要如下,点击左下角“阅读原文”可以自由获取论文PDF。

High-throughput density functional perturbation theory and machine learning predictions of infrared, piezoelectric, and dielectric responses
Kamal Choudhary, Kevin F. Garrity, Vinit Sharma, Adam J. Biacchi, Angela R. Hight Walker & Francesca Tavazza
Many technological applications depend on the response of materials to electric fields, but available databases of such responses are limited. Here, we explore the infrared, piezoelectric, and dielectric properties of inorganic materials by combining high-throughput density functional perturbation theory and machine learning approaches. We compute Γ-point phonons, infrared intensities, Born-effective charges, piezoelectric, and dielectric tensors for 5015 non-metallic materials in the JARVIS-DFT database. We find 3230 and 1943 materials with at least one far and mid-infrared mode, respectively. We identify 577 high-piezoelectric materials, using a threshold of 0.5 C/m2. Using a threshold of 20, we find 593 potential high-dielectric materials. Importantly, we analyze the chemistry, symmetry, dimensionality, and geometry of the materials to find features that help explain variations in our datasets. Finally, we develop high-accuracy regression models for the highest infrared frequency and maximum Born-effective charges, and classification models for maximum piezoelectric and average dielectric tensors to accelerate discovery.

扩展阅读
本文系网易新闻·网易号“各有态度”特色内容
媒体转载联系授权请看下方
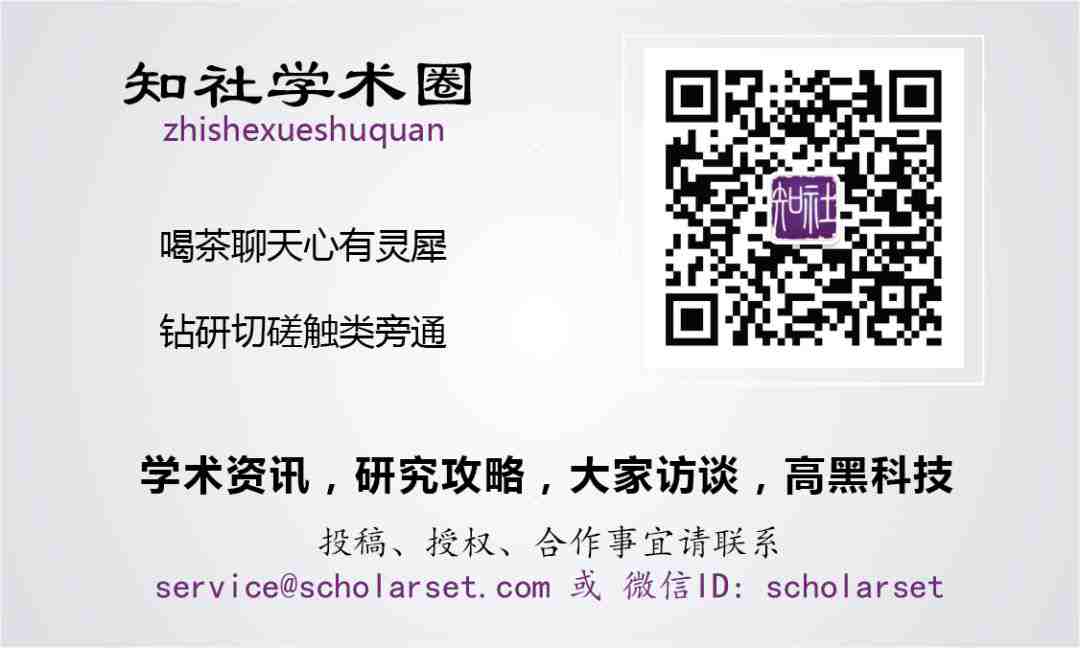
关键词
网易
模型
介电
机器学习
数据库
最新评论
推荐文章
作者最新文章
你可能感兴趣的文章
Copyright Disclaimer: The copyright of contents (including texts, images, videos and audios) posted above belong to the User who shared or the third-party website which the User shared from. If you found your copyright have been infringed, please send a DMCA takedown notice to [email protected]. For more detail of the source, please click on the button "Read Original Post" below. For other communications, please send to [email protected].
版权声明:以上内容为用户推荐收藏至CareerEngine平台,其内容(含文字、图片、视频、音频等)及知识版权均属用户或用户转发自的第三方网站,如涉嫌侵权,请通知[email protected]进行信息删除。如需查看信息来源,请点击“查看原文”。如需洽谈其它事宜,请联系[email protected]。
版权声明:以上内容为用户推荐收藏至CareerEngine平台,其内容(含文字、图片、视频、音频等)及知识版权均属用户或用户转发自的第三方网站,如涉嫌侵权,请通知[email protected]进行信息删除。如需查看信息来源,请点击“查看原文”。如需洽谈其它事宜,请联系[email protected]。