npj:机器学习——于一斑而窥压电体全豹

海归学者发起的公益学术平台
分享信息,整合资源
交流学术,偶尔风月
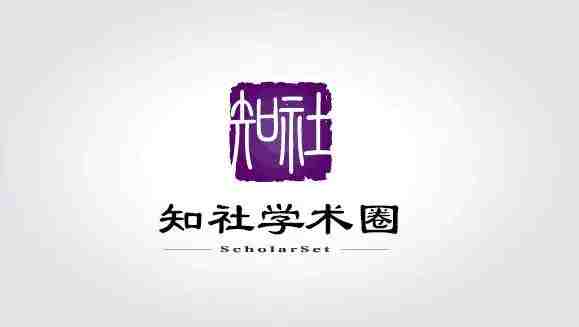
相图用作材料行为和材料属性的描述符,其形式与驱动系统的基础物理学紧密相连。尽管可以测量铁电到顺电相的转变温度,但这只适用于有限数量的数据点。采用局部探针无法完全获得任意组分和任意条件下压电体的功能响应特性。
现在,来自美国橡树岭国家实验室的Rama Vasudevan及其领导的波多黎各、美国和印度的研究人员研究表明,可用机器学习来获得完整的铁电相图。他们通过局部电滞回线将弛豫-铁电体系的相图映射为温度和材料组成的函数,以电压谱数据代表材料(未知的)微观状态参数或热力学参数;考虑到测量点的离散性,他们采用高斯过程来重构温度和电压空间中的电滞回线。将重构结果与原始数据和体介电谱测量数据进行比较,证实采用高斯过程的机器学习技术能够重建压电特性,从而结合常用方法即可准确预测铁电相图。这一技术可用于为功能材料自动绘制相图,并可筛选出最佳性能的材料组成,从而指导所需材料的合成。该文近期发表于npj Computational Materials 4: 23 (2018); doi:10.1038 /s41524-018-0078-7。英文标题与摘要如下,点击阅读原文可以自由获取论文PDF。

Reconstructing phase diagrams from local measurements via Gaussian processes: mapping the temperature-composition space to confidence
Dhiren K.Pradhan, Shalini Kumari, Evgheni Strelcov, Dillip K.Pradhan, Ram S. Katiyar, Sergei V.Kalinin, Nouamane Laanait & Rama K.Vasudevan
We show the ability to map the phase diagram of a relaxor-ferroelectric system as a function of temperature and composition through local hysteresis curve acquisition, with the voltage spectroscopy data being used as a proxy for the (unknown) microscopic state or thermodynamic parameters of materials. Given the discrete nature of the measurement points, we use Gaussian processes to reconstruct hysteresis loops in temperature and voltage space, and compare the results with the raw data and bulk dielectric spectroscopy measurements. The results indicate that the surface transition temperature is similar for all but one composition with respect to the bulk. Through clustering algorithms, we recreate the main features of the bulk diagram, and provide statistical confidence estimates for the reconstructed phase transition temperatures. We validate the method by using Gaussian processes to predict hysteresis loops for a given temperature for a composition unseen by the algorithm, and compare with measurements. These techniques can be used to map phase diagrams from functional materials in an automated fashion, and provide a method for uncertainty quantification and model selection.

本文系网易新闻·网易号“各有态度”特色内容
媒体转载联系授权请看下方
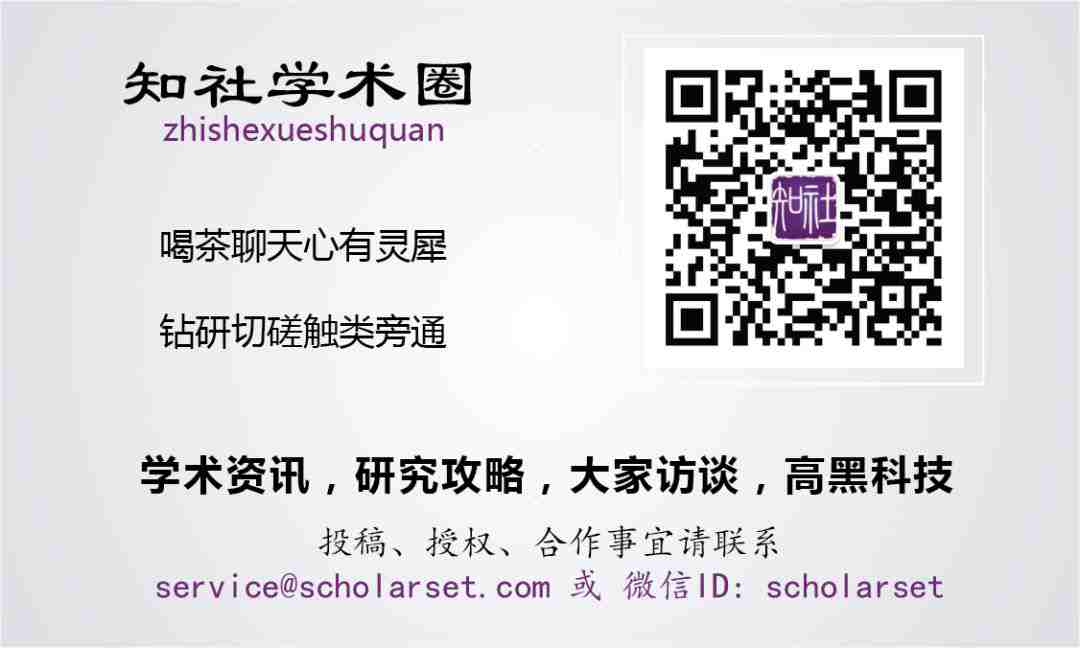
最新评论
推荐文章
作者最新文章
你可能感兴趣的文章
Copyright Disclaimer: The copyright of contents (including texts, images, videos and audios) posted above belong to the User who shared or the third-party website which the User shared from. If you found your copyright have been infringed, please send a DMCA takedown notice to [email protected]. For more detail of the source, please click on the button "Read Original Post" below. For other communications, please send to [email protected].
版权声明:以上内容为用户推荐收藏至CareerEngine平台,其内容(含文字、图片、视频、音频等)及知识版权均属用户或用户转发自的第三方网站,如涉嫌侵权,请通知[email protected]进行信息删除。如需查看信息来源,请点击“查看原文”。如需洽谈其它事宜,请联系[email protected]。
版权声明:以上内容为用户推荐收藏至CareerEngine平台,其内容(含文字、图片、视频、音频等)及知识版权均属用户或用户转发自的第三方网站,如涉嫌侵权,请通知[email protected]进行信息删除。如需查看信息来源,请点击“查看原文”。如需洽谈其它事宜,请联系[email protected]。