机器学习增强X射线视野,加速材料基因设计
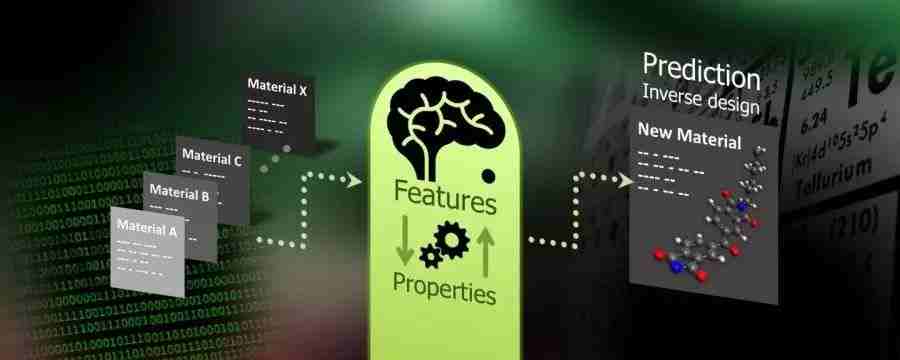
海归学者发起的公益学术平台
分享信息,整合资源
交流学术,偶尔风月
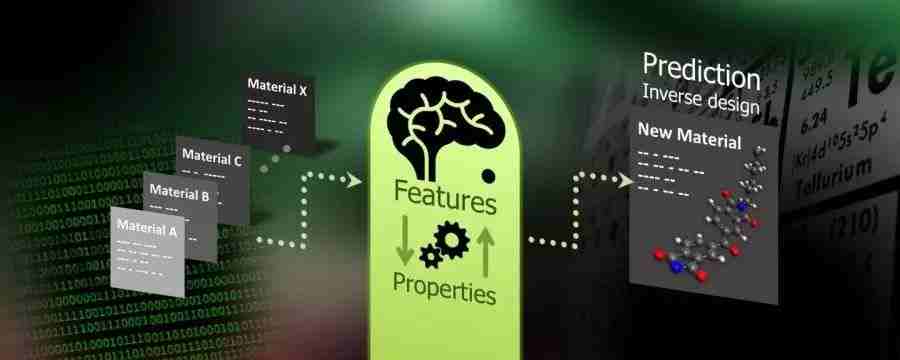
机器学习在管理全方位不断增长的高通量材料研究数据方面,拥有难以估量的作用,特别是用于快速自动识别高通量表征数据背后隐含的潜在新材料的成分相图,推进新材料的发现和制备-结构-性能一体化分析。当新型合金含有三种或更多的金属元素时,不同元素成分的组合将带来大量结构和性能各异的材料,为合金设计与合成带来巨大的挑战。通过机器学习和人工智能自动分析这些材料海量的X光衍射数据,则可能帮助加速并提升新型多元合金的设计与开发。来自美国国家标准局的A. Gilad Kusne及其同事的研究显示,通过机器学习技术,可以量化不同材料的关键结构数据,如X-射线衍射峰强度和位置及其随样品组分的变化,进而加速新型材料的研发过程。为证实这一思路,该研究小组制备了成分分散的铁-钴-镍合金薄膜,然后运用不同软件分析其X射线的异同,评估不同算法的处理速度和准确性。最终,他们找到几种算法,适用于高通量生成彩色编码图样,在二维和三维层面展示合金成分和物相之间的关系。这一工作,近日已发表在npj Computational Materials上,英文摘要及原文链接如下,文末点击“阅读原文”可以自由下载论文PDF。

Comparison of dissimilarity measures for cluster analysis of X-ray diffraction data from combinatorial libraries
Yuma Iwasaki, A. Gilad Kusne & Ichiro Takeuchi
Machine learning techniques have proven invaluable to manage the ever growing volume of materials research data produced as developments continue in high-throughput materials simulation, fabrication, and characterization. In particular, machine learning techniques have been demonstrated for their utility in rapidly and automatically identifying potential composition–phase maps from structural data characterization of composition spread libraries, enabling rapid materials fabrication-structure-property analysis and functional materials discovery. A key issue in development of an automated phase-diagram determination method is the choice of dissimilarity measure, or kernel function. The desired measure reduces the impact of confounding structural data issues on analysis performance. The issues include peak height changes and peak shifting due to lattice constant change as a function of composition. In this work, we investigate the choice of dissimilarity measure in X-ray diffraction-based structure analysis and the choice of measure’s performance impact on automatic composition-phase map determination. Nine dissimilarity measures are investigated for their impact in analyzing X-ray diffraction patterns for a Fe–Co–Ni ternary alloy composition spread. The cosine, Pearson correlation coefficient, and Jensen–Shannon divergence measures are shown to provide the best performance in the presence of peak height change and peak shifting (due to lattice constant change) when the magnitude of peak shifting is unknown. With prior knowledge of the maximum peak shifting, dynamic time warping in a normalized constrained mode provides the best performance. This work also serves to demonstrate a strategy for rapid analysis of a large number of X-ray diffraction patterns in general beyond data from combinatorial libraries.

文末点击“阅读原文”可以自由下载论文PDF。
如需转载或者合作请看下方↓↓↓
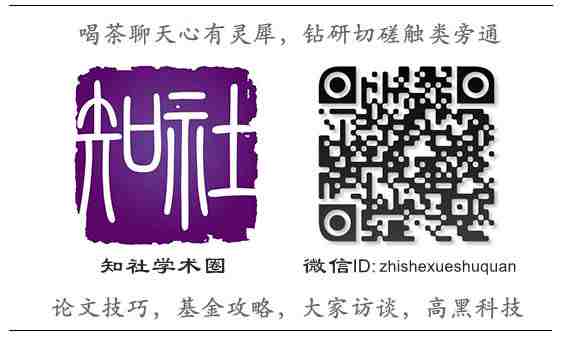
[email protected] 或微信ID: scholarset
回复“目录”或“分类”,浏览知社更多精华。长按二维码识别,可以关注/进入公众号进行回复。
最新评论
推荐文章
作者最新文章
你可能感兴趣的文章
Copyright Disclaimer: The copyright of contents (including texts, images, videos and audios) posted above belong to the User who shared or the third-party website which the User shared from. If you found your copyright have been infringed, please send a DMCA takedown notice to [email protected]. For more detail of the source, please click on the button "Read Original Post" below. For other communications, please send to [email protected].
版权声明:以上内容为用户推荐收藏至CareerEngine平台,其内容(含文字、图片、视频、音频等)及知识版权均属用户或用户转发自的第三方网站,如涉嫌侵权,请通知[email protected]进行信息删除。如需查看信息来源,请点击“查看原文”。如需洽谈其它事宜,请联系[email protected]。
版权声明:以上内容为用户推荐收藏至CareerEngine平台,其内容(含文字、图片、视频、音频等)及知识版权均属用户或用户转发自的第三方网站,如涉嫌侵权,请通知[email protected]进行信息删除。如需查看信息来源,请点击“查看原文”。如需洽谈其它事宜,请联系[email protected]。