Rust解码Protobuf数据比Go慢五倍?记一次性能调优之旅
平均阅读时长为 2分钟
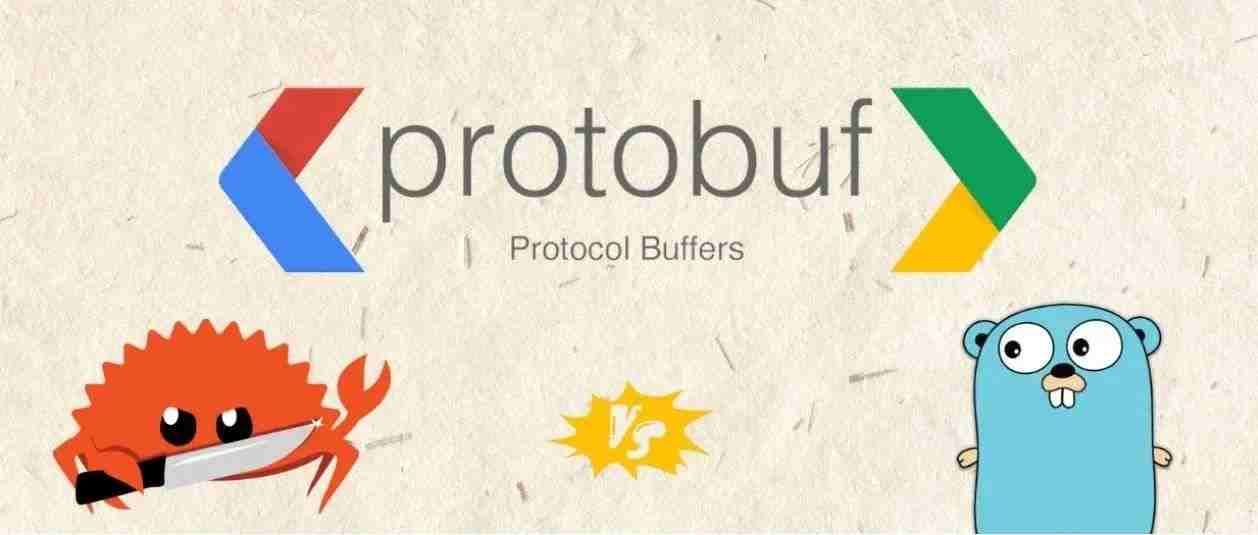
为了简化行文,所有的测试代码存放在 GitHub 仓库中:
https://github.com/v0y4g3r/prom-write-request-bench
git clone https://github.com/v0y4g3r/prom-write-request-bench
cd prom-write-request-bench
export PROJECT_ROOT=$(pwd)
首先我们尝试使用一个最小可复现的 benchmark 来确认此问题。
git checkout step1/reproduce
fnbench_decode_prom_request(c: &mut Criterion) {
letmut d = std::path::PathBuf::from(env!("CARGO_MANIFEST_DIR"));
d.push("assets");
d.push("1709380533560664458.data");
letdata = Bytes::from(std::fs::read(d).unwrap());
letmut request_pooled = WriteRequest::default();
c.benchmark_group("decode")
.bench_function("write_request", |b| {
b.iter(|| {
letmut request = WriteRequest::default();
letdata = data.clone();
request.merge(data).unwrap();
});
});
}
cargo bench -- decode/write_request
decode/write_request
time: [7.3174 ms 7.3274 ms 7.3380 ms]
change: [+128.55% +129.11% +129.65%] (p = 0.00 < 0.05)
git clone https://github.com/VictoriaMetrics/VictoriaMetrics
cd VictoriaMetrics
cat <<EOF > ./lib/prompb/prom_decode_bench_test.go
package prompb
import (
"io/ioutil"
"testing"
)
func BenchmarkDecodeWriteRequest(b *testing.B) {
data, _ := ioutil.ReadFile("${PROJECT_ROOT}/assets/1709380533560664458.data")
wr := &WriteRequest{}
for n := 0; n < b.N; n++ {
b.StartTimer()
wr.Reset()
err := wr.UnmarshalProtobuf(data)
if err != nil {
panic("failed to unmarshall")
}
b.StopTimer()
}
}
EOF
go test github.com/VictoriaMetrics/VictoriaMetrics/lib/prompb --bench BenchmarkDecodeWriteRequest
🌟 Go 版本中,数据文件路径指向 prom-write-request-bench 代码仓库的 assets 目录下的 1709380533560664458.data 文件。
goos: linux
goarch: amd64
pkg: github.com/VictoriaMetrics/VictoriaMetrics/lib/prompb
cpu: AMD Ryzen 7 7735HS with Radeon Graphics
BenchmarkDecodeWriteRequest-16 961 1196101 ns/op
PASS
ok github.com/VictoriaMetrics/VictoriaMetrics/lib/prompb 1.328s
可以看到 Rust 解析一个 10000 条时间线的 Prometheus 写入请求耗费了 7.3ms 左右,而 VictoriaMetrics 的 Go 版本只花费了 1.2ms,仅为 Rust 版本的 1/6。
聪明的你可能一下子就看到问题所在了。在 Go 版本中,每次反序列化都是使用的相同的 WriteRequest 结构体,只是在反序列化之前执行 reset 避免数据污染而已,而 Rust 这边每次反序列化都会使用一个新的结构体。
这正是 VictoriaMetrics 为写入性能所做的优化之一。VictoriaMetrics 的写入路径上大量使用对象池化(sync.Pool)技术来降低垃圾回收的压力。假设 Go 版本也像 Rust 一样每次构建一个新的结构体用于反序列化,那么 Go 版本的耗时将会增加到 10ms 左右,比上面测出的 Rust 的结果要差。
那么在 Rust 版本中可以使用类似的池化技术吗?
let mut request_pooled = WriteRequest::default();
c.bench_function("pooled_write_request", |b| {
b.iter(|| {
let data = data.clone();
request_pooled.clear();
request_pooled.merge(data).unwrap();
});
});
然后执行:
cargo bench -- decode/pooled_write_request
decode/pooled_write_request
time: [7.1445 ms 7.1645 ms 7.1883 ms]
可以看到性能并没有特别大的提升。那么这是为什么呢?
🌟 当前性能对比回顾
- Rust 基线耗时:7.3ms
- Go 解析耗时:1.2ms
- Rust 当前耗时:7.1ms
git checkout step2/repeated_field
(Prometheus 的 WriteRequest 的数据结构图)
WriteRequest 持有一个 TimeSeries 的 vector,而每个 TimeSeries 又分别持有 Label ,Sample 和 Examplar 的 vector。如果我们仅仅复用最外层的 WriteRequest,每次 clear 的时候,Labels,Samples 和 Examplars 的 vector 都会被清除,根本达不到复用的目的。
wr.Timeseries = tss[:0]
并非是将 TimeSeries 字段设置为 nil,而是设置为一个空 slice,那么 slice 当中原有的元素仍然会被保留而不会被 GC(只是 len 字段设置为了 0 而已),因此 Go 的对象复用机制可以取得很好的避免反复分配内存的效果。
那么 Rust 有没有可能借鉴这个机制捏?
我们想到了 Rust 生态中另一个较为常用的 Protobuf 库 rust-protobuf v2.x 版本的一个机制:RepeatedField[3]。其设计为了避免 Vec::clear 带来的 drop 开销,手动维护了 vec 和 len 字段,在 clear 时仅仅把 len 设为 0 而不调用 vec 的 clear,从而保证 vec 里面的元素以及这些元素内部的 vec 不被 drop。
那么问题来了,怎么把 RepeatedField 的机制集成到 PROST 里面呢?显然 PROST 是没有类似的配置项的,那么就需要我们把 PROST 的过程宏生成的代码手动展开了。
cargo bench -- decode/pooled_write_request
decode/pooled_write_request
time: [2.6941 ms 2.7004 ms 2.7068 ms]
change: [-66.969% -66.417% -65.965%] (p = 0.00 < 0.05)
Performance has improved.
Wow,我们通过 RepeatedField 机制成功将耗时降到了原先的 36% 左右。
那么这个耗时是否还能进一步降低呢?我们还能从 Go 的代码中学到什么?
值得一提的是,由于 RepeatedField 在使用上不如 Vec 方便,因此 rust-protobuf 的 3.x 版本已经去除了[4]。不过 作者也提到过[5] 可能会提供一个选项再把 RepeatedField 加回来。
🌟 当前性能对比回顾
- Rust 基线耗时:7.3ms
- Go 解析耗时:1.2ms
- Rust 当前耗时:2.7ms
git checkout step3/bytes
众所周知,Go 中的 string 只是 bytes 的一个简单包装,反序列化 string 字段时只需要把原始 buffer 的指针和长度赋值给 string 字段即可。而 Rust 的 PROST 在反序列化 String 类型的字段时,需要将原始 buffer 中的数据复制到 String 中去,这样才能保证反序列化之后的结构体的生命周期和原始的 buffer 相互独立。但是这样就多了一次数据复制的开销。
我们是否也可以把 Label 的字段改为 Bytes 而不是 String 呢?我想起了 prost_build 中有个 Config::bytes 选项[6]。在 PROST 的这个 PR 中支持将 bytes类型的字段生成为 Bytes而不是默认的 Vec<u8>从而可以实现零拷贝的解析[7]。我们同样可以将 Label 的 name 和 value 字段类型改为 Bytes。这样做的优点是无需复制,但是问题也很明显,在需要使用 Label 的地方还是需要把 Bytes 转换为 String 。在转换这个步骤中,我们可以选择 String::from_utf8_unchecked 来跳过字符串是否合法的检查从而进一步提高性能。当然如果 GreptimeDB 实例暴露在公网中这样的操作显然是不安全的,因此在 #3435[8] 中我们提到了需要增加一个严格模式来校验字符串是否合法。
cargo bench -- decode/pooled_write_request
decode/pooled_write_request
time: [3.4295 ms 3.4315 ms 3.4336 ms]
change: [+26.763% +27.076% +27.383%] (p = 0.00 < 0.05)
Performance has regressed.
(火焰图结果显示)
可以看到,绝大多数的 CPU 时间片都耗费在了 copy_to_bytes 上面。
pubfnmerge<A, B>(
wire_type: WireType,
value: &mut A,
buf: &mut B,
_ctx: DecodeContext,
) -> Result<(), DecodeError>
where
A: BytesAdapter,
B: Buf,
{
check_wire_type(WireType::LengthDelimited, wire_type)?;
letlen = decode_varint(buf)?;
if len > buf.remaining() asu64 {
returnErr(DecodeError::new("buffer underflow"));
}
letlen = len asusize;
//...
value.replace_with(buf.copy_to_bytes(len));
Ok(())
}
implsealed::BytesAdapter forBytes {
...
fnreplace_with<B>(&mutself, mut buf: B)
where
B: Buf,
{
*self = buf.copy_to_bytes(buf.remaining());
}
...
}
那我们是否可以省略一次 copy 呢?虽然说 Bytes::copy_to_bytes 并不涉及到数据的复制,只是指针操作,但是其开销还是比较大的。
🌟 当前性能对比回顾
- Rust 基线耗时:7.3ms
- Go 解析耗时:1.2ms
- Rust 当前耗时:3.4ms
git checkout step4/bytes-eliminate-one-copy
#[inline(always)]
fncopy_to_bytes(data: &mut Bytes, len: usize) -> Bytes {
if len == data.remaining() {
std::mem::replace(data, Bytes::new())
} else {
letret = data.slice(0..len);
data.advance(len);
ret
}
}
pubfnmerge_bytes(value: &mut Bytes, buf: &mut Bytes) -> Result<(), DecodeError> {
letlen = decode_varint(buf)?;
if len > buf.remaining() asu64 {
returnErr(DecodeError::new(format!(
"buffer underflow, len: {}, remaining: {}",
len,
buf.remaining()
)));
}
*value = copy_to_bytes(buf, len asusize);
Ok(())
}
cargo bench -- decode/pooled_write_request
decode/pooled_write_request
time: [2.7597 ms 2.7630 ms 2.7670 ms]
change: [-19.582% -19.483% -19.360%] (p = 0.00 < 0.05)
Performance has improved.
有进步,但不多,好像只是又回到刚刚的性能水平了。那么能不能再进一步呢?
🌟 当前性能对比回顾
- Rust 基线耗时:7.3ms
- Go 解析耗时:1.2ms
- Rust 当前耗时:2.76ms
对应分支:
git checkout step5/bench-bytes-slice
首先我们看看,为什么 PROST 需要做两次 copy 呢?
主要是因为 PROST 的字段的 trait bound 是 BytesAdapter 而反序列化的 Bytes 的 trait bound 则是 Buf。虽然 Bytes 同时实现了两个 trait ,但是如果两个类型相互赋值,还是需要走 copy_to_bytes 转换两次。
(Merge 方法的转换实现)
从 Prost 角度来看,Bytes::copy_to_bytes 并不涉及到数据的复制,因此可以看做是一个 zero copy 的操作。但是这个 zero copy 操作的开销并没有那么低。
c.benchmark_group("slice").bench_function("bytes", |b| {
letmut data = data.clone();
b.iter(|| {
letmut bytes = data.clone();
for_in0..10000 {
bytes = black_box(bytes.slice(0..1));
}
});
});
funcBenchmarkBytesSlice(b *testing.B) {
data, _ := ioutil.ReadFile("<any binary file>")
for n := 0; n < b.N; n++ {
b.StartTimer()
bytes := data
for i :=0; i < 10000; i++ {
bytes = bytes[:1]
}
b.StopTimer()
}
}
goos: linux
goarch: amd64
pkg: github.com/VictoriaMetrics/VictoriaMetrics/lib/prompb
cpu: AMD Ryzen 7 7735HS with Radeon Graphics
BenchmarkBytesSlice-16 497607 2930 ns/op
PASS
ok github.com/VictoriaMetrics/VictoriaMetrics/lib/prompb 6.771s
slice/bytes
time: [103.23 µs 103.31 µs 103.40 µs]
change: [+7.6697% +7.8029% +7.9374%] (p = 0.00 < 0.05)
可以看到 slice 这个操作 Rust 比 Go 慢了 2 个数量级。
(slice 的操作示意图)
(Bytes 引用计数机制)
- 通过 From<Vec<u8>> 构造出来的的 Bytes 的 slice 操作基于引用计数,每次 slice 的时候需要复制原始 buffer 的指针、长度、引用计数、slice 返回值的指针和长度等等,和 Go 的基于可达性分析的垃圾回收机制比起来效率肯定是差很多的;
- 由于 Bytes 支持多种实现,部分方法(如 clone)依赖 vtable 进行动态分发[11];
- 为了确保 slice 操作的安全性,Bytes 在很多地方手动插入了 bound check[12]。
git checkout step6/optimize-slice
(Bytes 实例解析)
pubfnsplit_to(buf: &mut Bytes, end: usize) -> Bytes {
letlen = buf.len();
assert!(
end <= len,
"range end out of bounds: {:?} <= {:?}",
end,
len,
);
if end == 0 {
return Bytes::new();
}
letptr = buf.as_ptr();
letx = unsafe { slice::from_raw_parts(ptr, end) };
// `Bytes::drop` does nothing when it's built via `from_static`.
Bytes::from_static(x)
}
//.benchmark
c.bench_function("split_to", |b| {
letdata = data.clone();
b.iter(|| {
letmut bytes = data.clone();
for_in0..10000 {
bytes = black_box(unsafe { split_to(&bytes, 1) });
}
});
})
再 Bench 一下看看效果:
slice/bytes
time: [103.23 µs 103.31 µs 103.40 µs]
change: [+7.6697% +7.8029% +7.9374%] (p = 0.00 < 0.05)
slice/split_to
time: [24.061 µs 24.089 µs 24.114 µs]
change: [+0.2058% +0.4198% +0.6371%] (p = 0.00 < 0.05)
decode/pooled_write_request
time: [1.6169 ms 1.6181 ms 1.6193 ms]
change: [-37.960% -37.887% -37.815%] (p = 0.00 < 0.05)
Performance has improved.
终于,我们可以把解析单个 WriteRequest 的耗时降低到了 1.6ms 左右,只比 Go 的 1.2ms 慢 33.3% 了呢!
,可以达到接近 Go 的性能(仅考虑 slice 的开销):
c.bench_function("slice", |b| {
let data = data.clone();
let mut slice = data.as_ref();
b.iter(move || {
for _ in 0..10000 {
slice = black_box(&slice[..1]);
}
});
});
slice/slice
time: [4.6192 µs 4.7333 µs 4.8739 µs]
change: [+6.1294% +9.8655% +13.739%] (p = 0.00 < 0.05)
Performance has regressed.
但是由于这部分开销在整个写入链路中占比已经非常低,再继续优化对整体吞吐的影响并不会很大,感兴趣的小伙伴可以自行尝试一下使用 slice 重构反序列化的代码。
🌟 当前性能对比回顾
- Rust 基线耗时:7.3ms
- Go 解析耗时:1.2ms
- Rust 当前耗时:1.62ms
Reference:
[1] https://github.com/prometheus/prometheus/blob/main/prompb/remote.proto#L22-L28
[2] https://github.com/VictoriaMetrics/VictoriaMetrics/blob/c005245741fc3d7d744f258959be2a5ae388f8ec/lib/prompb/prompb.go#L19-L37
[3]https://docs.rs/protobuf/2.28.0/protobuf/struct.RepeatedField.html
[4] https://github.com/stepancheg/rust-protobuf/issues/518#issuecomment-751870333
[5] https://github.com/stepancheg/rust-protobuf/issues/503#issuecomment-1030822294
[6] https://docs.rs/prost-build/latest/prost_build/struct.Config.html#method.bytes
[7] https://github.com/tokio-rs/prost/pull/341
[8] https://github.com/GreptimeTeam/greptimedb/issues/3435
[9] https://github.com/tokio-rs/prost/blob/0bd94826f04b7ead7dc6a0ad2ac749374cd2dfd3/src/encoding.rs#L989
[10] https://github.com/tokio-rs/prost/blob/0bd94826f04b7ead7dc6a0ad2ac749374cd2dfd3/src/encoding.rs#L912
[11] https://docs.rs/bytes/1.5.0/src/bytes/bytes.rs.html#529-534
[12] https://docs.rs/bytes/1.5.0/src/bytes/bytes.rs.html#255-266
[13] https://github.com/GreptimeTeam/greptimedb/pull/3425
[14] https://github.com/GreptimeTeam/greptimedb/pull/3478
- GreptimeDB 是一款用 Rust 语言编写的时序数据库,具有分布式、开源、云原生、兼容性强等特点,帮助企业实时读写、处理和分析时序数据的同时,降低长期存储的成本。
- GreptimeCloud 基于开源的 GreptimeDB,为用户提供全托管的 DBaaS,能够与可观测性、物联网等领域结合的应用产品结合。利用云提供软件和服务,可以达到快速的自助开通和交付,标准化的运维支持,和更好的资源弹性。现已正式公测,欢迎关注公众号或官网了解最新动态!
- GreptimeAI 是为 LLM 应用量身定制的可观测性解决方案,开发者可以通过该方案全面、深入地了解应用的成本、性能、流量和安全情况,在保证低成本和高性能的同时提供高效可靠的分析能力,同时保留了时序数据库的灵活性。
- 车云一体解决方案 是一款深入车企实际业务场景的时序数据库解决方案,解决了企业车辆数据呈几何倍数增长后的实际业务痛点。
GreptimeDB 作为开源项目,欢迎对时序数据库、Rust 语言等内容感兴趣的同学们参与贡献和讨论。第一次参与项目的同学推荐先从带有 Good First Issue 标签的 Issue 入手,期待在开源社群里遇见你!
Star us on GitHub Now:
https://github.com/GreptimeTeam/greptimedb
官网:https://greptime.cn/
文档:https://docs.greptime.cn/
Twitter: https://twitter.com/Greptime
Slack: https://greptime.com/slack
LinkedIn: https://www.linkedin.com/company/greptime/
最新评论
推荐文章
作者最新文章
你可能感兴趣的文章
Copyright Disclaimer: The copyright of contents (including texts, images, videos and audios) posted above belong to the User who shared or the third-party website which the User shared from. If you found your copyright have been infringed, please send a DMCA takedown notice to [email protected]. For more detail of the source, please click on the button "Read Original Post" below. For other communications, please send to [email protected].
版权声明:以上内容为用户推荐收藏至CareerEngine平台,其内容(含文字、图片、视频、音频等)及知识版权均属用户或用户转发自的第三方网站,如涉嫌侵权,请通知[email protected]进行信息删除。如需查看信息来源,请点击“查看原文”。如需洽谈其它事宜,请联系[email protected]。
版权声明:以上内容为用户推荐收藏至CareerEngine平台,其内容(含文字、图片、视频、音频等)及知识版权均属用户或用户转发自的第三方网站,如涉嫌侵权,请通知[email protected]进行信息删除。如需查看信息来源,请点击“查看原文”。如需洽谈其它事宜,请联系[email protected]。